DHS ARI IWAS introduced its 5th webinar on January 27, 2022 teaching undergraduate and graduate students the fundamentals of Principal Component Analysis (PCA).
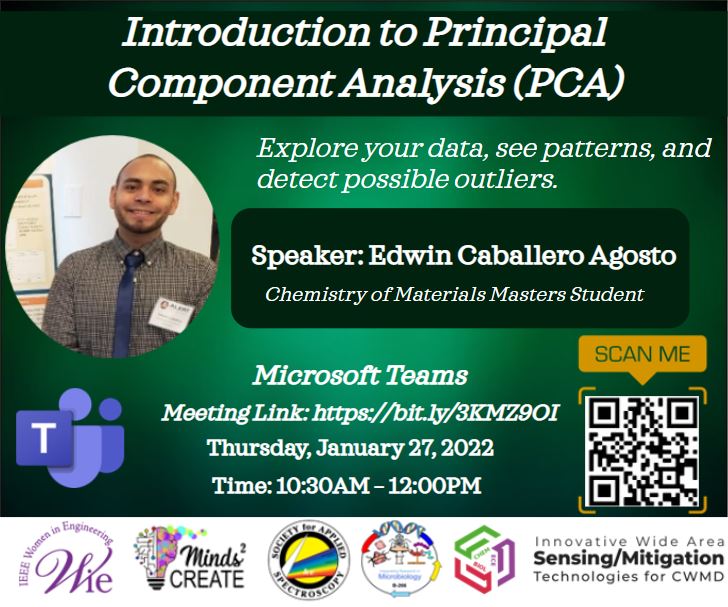
Principal Component Analysis (PCA) is a statistical method that is used to reduce the dimensionality of data. PCA is a type of linear transformation that projects the data onto a lower-dimensional space, such that the maximum amount of variance is preserved. This can be useful for visualizing the data, for removing noise and outliers, and for reducing the computational complexity of data analysis.
PCA is commonly used in a variety of fields, including machine learning, computer vision, and chemometrics. In chemometrics, PCA is often used to analyze spectral data, such as infrared or Raman spectra. PCA can help researchers to identify the most important features in the data, to remove noise and outliers, and to visualize the data in a more meaningful way. It can also be used to reduce the dimensionality of the data, which can improve the performance of other data analysis methods, such as clustering or classification.
Overall, PCA is a powerful and versatile tool for data analysis, and can be useful for researchers in a wide range of fields. It can help to simplify complex data sets, to identify important features, and to improve the accuracy and interpretability of data analysis.